Popular scientific abstract
The development of transportation has greatly benefited our lives, however, traffic crashes can be also a threat to people’s life. Many safety systems have been developed to prevent traffic crashes and mitigate crash injuries. Plenty of driving data for manually driven cars have been collected and implemented in virtual simulation for safety benefit studies. Safety assessments of manually driven cars with and without safety systems have also been studied during past years. However, even though automated vehicles will be the future trend, there is no established safety assessment method available for automated vehicles interactions with pedestrians and bicyclists. There is limited automated vehicle driving data available and real-life experiment of automated vehicles can also face many difficulties. Automated vehicles can potentially improve (e.g., on-line software updates) or learn themselves from previous driving experience, which leads to more uncertainties in data collection. In addition to the lack of established safety assessment method, there is also a lack of robust statistical methods to use in conjunction with virtual assessment of safety. This project will address these two gaps and when this project come to the end, we will have better understanding of the influence of pedestrians and bicyclists interactions with automated vehicles, regarding the crash risks.
My affiliation
Supervisor contacts:
Jonas Bärgman: jonas.bargman@chalmers.se
Carol Flannagan (co-supervisor; UMTRI): andras.balint@chalmers.se
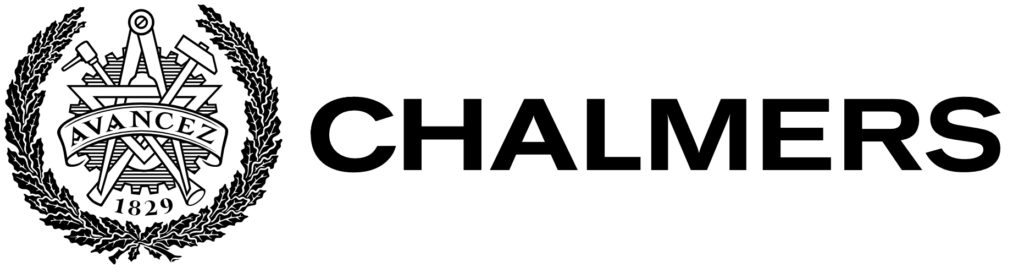
Background
As automated vehicles are to be introduced in traffic there is a clear and present need to evaluate the automated vehicles functionalities before the systems are running in production vehicles on our roads. To do this timely (e.g., during development or between software releases), virtual safety assessment is needed. Virtual simulations for safety benefit assessment has been increasingly popular and broadly implemented in last few decades (Lindman & Tivesten, 2006; Sander, 2017). Although there is ongoing research on methods for virtual assessment of traffic safety between automated cars and other road-users (Wang et al., 2020), there are several research gaps. The following are the two research gaps which this PhD will address: a) a lack of methods for virtually assessing the safety of automated vehicle interaction with pedestrians and bicyclists, b) a lack of robust statistical methods to use in conjunction with virtual assessment of safety, and that can use prior information and merge data from multiple data sources. For the former, continuation of current methods, but integrating models of pedestrian and bicyclist behavior models, is a next natural research step. For the latter, the use of Bayesian statistics as a framework to include prior information has been identified as a promising direction of research, but with very little prior work available.
Aims and objectives
Aims:
The aim of this PhD project is a) to train the (Marie Curie) PhD on a wide variety of methods and theories within the field of human factors for vehicle automation in the future, with exposure also to industry, and b) to develop a method for virtually assessing the safety benefits of automated vehicles interaction with vulnerable road users (VRU; bicyclists and pedestrians) in an appropriate statistical framework, for example Bayesian, integrating computational models of human-interaction with automated vehicles into virtual counterfactual simulations as input to the framework.
Objectives:
- Develop and improve methods for the virtual assessment of the crash risks associated with the combination of the AV driver, the AV, and human road users (e.g., VRUs) interacting with it.
- Develop method components needed for virtual assessment, with focus on sampling methods for testing, and crash (baseline) generation considering human behaviours
- • Compare the safety (crash risk) of AV functionalities and manually-driven cars across different scenarios involving VRUs, taking into account VRU behaviour
Research description
Together with 14 other ESRs, we will be trained and performing research on human factors aspects of the interaction between automated vehicles and humans in the cities of tomorrow in the Marie Curie project SHAPE-IT. Specifically, I will be performing research to develop and evaluate methods for virtually assessing the safety benefit of automated vehicles when interacting with vulnerable road users (VRU; pedestrians and bicyclists). The project has two main parts. First, the work includes the development of a simulation framework (concept and implementation of counterfactual simulations) to assess different car/VRU conflict situations. Second, the other main part of the work is to develop a statistical/methodological framework, for example using Bayesian statistics that uses the virtual simulations and prior knowledge about system benefits (and other aspects of the conflicts). The work will include the comparison of such a Bayesian framework and traditional statistical methods
Results
The following is an overview of Xiaomi’s work in relation to the objectives and expected results.
The first two objectives target the same aspect of virtual simulations for safety assessment – improved methods. Xiaomi has pursued this from two main perspectives – both related to which data to use. She has performed research on a novel method (and its application in the traffic safety domain) to reduce the resources/time it takes to complete virtual safety assessments with multi-variate distributions. Specifically, together with another PhD student she developed (and provided demonstration of) a method the call active sampling, which use machine learning methods to help to optimize parameter sampling in virtual assessment and available here as a pre-print. A follow-up paper is almost completed, further extending the method and provide a deeper demonstration of its use in the vehicle safety domain.
Xiaomi also developed a method called predictive Bayesian optional stopping (pBOS), building on work by the statistician Kruschke (see for example his book “Doing Bayesian data analysis”, 2014). The method enables studies to be stopped early if it is not likely that the study will reach the sought target with the (predetermined) available resources. That is, it is a form of predictive Bayesian power analysis, that is likely to reduce the cost of studies (especially in industry, as they can stop experiments that they realize will not provide results early). This work is to be submitted in May or June 2024.
Xiaomi further performed a virtual simulation study that investigated how automated emergency braking (a critical component in AVs) that includes considerations of human comfort zone boundaries (CZB) may improve safety for conflicts between cars and powered two-wheelers (PTWs). She found that AEB algorithms with a CZB component result in substantial safety improvements, compared to traditional AEB algorithms. More generally, this work indicates that including drivers’ CZBs in AV algorithms may improve safety performance, while reducing the risk for nuisance interventions and increasing trust.
Although the results of Xiaomi’s research likely will not directly lead to policymaking recommendations, the work is likely to help the automotive industry to reduce the resources needed to conduct both physical and virtual experiments (i.e., as part of virtual safety assessment), and to guide the developers of AVs towards using comfort zone boundary-based algorithms for improved safety performance while reducing driver nuisance.
My publications
Yang, X. (2023). Safety Evaluation Using Counterfactual Simulations: The use of computational driver behavior models in crash avoidance systems and virtual simulations with optimal subsampling (Licentiate thesis; Publication Number 2023:04) Chalmers University of Technology]. Gothenburg, Sweden. https://research.chalmers.se/publication/534653/file/534653_Fulltext.pdf
Yang, X., Lubbe, N., & Bärgman, J. Automated Emergency Braking algorithms based on comfort zone boundaries outperform traditional algorithm: Virtual benefit assessment for car-to-powered-two-wheeler crashes in China. (2024) IET Intelligent Transport Systems. (Second revision submitted, minor revision)
Imberg, H., Yang, X., Flannagan, C., & Bärgman, J. (2024). Active sampling: A machine-learning-assisted framework for finite population inference with optimal subsamples. Technometrics. (tentatively accepted; revised manuscript and replication code and data submitted in May 2024: original completion of the paper and resubmission delayed due to the main author being on parental leave; pre-print: https://arxiv.org/abs/2212.10024)
References and links
Lindman, M., & Tivesten, E. (2006). A method for estimating the benefit of autonomous braking systems using traffic accident data. SAE Technical Papers, 2006(724). https://doi.org/10.4271/2006-01-0473
Sander, U. (2017). Opportunities and limitations for intersection collision intervention—A study of real world ‘left turn across path’ accidents. Accident Analysis and Prevention. https://doi.org/10.1016/j.aap.2016.12.011
Wang, L., Zhong, H., Ma, W., Abdel-Aty, M., & Park, J. (2020). How many crashes can connected vehicle and automated vehicle technologies prevent: A meta-analysis. Accident Analysis and Prevention. https://doi.org/10.1016/j.aap.2019.105299